This tree risk assessment review article by Peter Gray, from the Summer 2020 issue of Arboriculture Australia's 'The Bark', might be of interest to you.
Disadvantages
Interestingly, both of VALID's
disadvantages in the article are in fact
advantages.
1) The 'mathematics professor' and the risk model
The 'mathematics professor' isn't a mathematics professor. His name's Willy Aspinall and he's the Cabot Professor in Natural Hazards & Risk Science at the University of Bristol. He's a 'risk professor' who we worked with when developing VALID's risk model that does the hard work behind the scenes in the App. He's driven the model to breaking point and this is what he has to say about it:
“We have stress tested VALID and didn’t find any gross, critical sensitivities. In short, the mathematical basis of your approach is sufficiently robust and dependable for any practical purpose.”
2) Risk overvaluation? - Death by numberwang
"The risk of harm* for incidents involving motor vehicles (not motor cycles) appears to be high. There is little evidence of people being killed from cars running into fallen trees but this still apparently has a significant input to the calculated risk of harm."
This gets a bit detailed. In short, the risk isn't too high in VALID's risk model when it comes to vehicles.
There's a couple of points here. First, VALID's risk model doesn't try to measure a death. Death is too narrow and accurate a consequence for a risk that has this much uncertainty. Here's the long answer, and we shared a version of this with Peter after reading his thoughtful article.
The essence of the conundrum with traffic is that it's seldom that a death occurs unless a tree or a large branch hits the cab space of a moving vehicle. So, how do you go about modelling the consequences when they're usually a vehicle driving into a tree during its stopping distance, rather than tree part hitting the relatively small cab space?
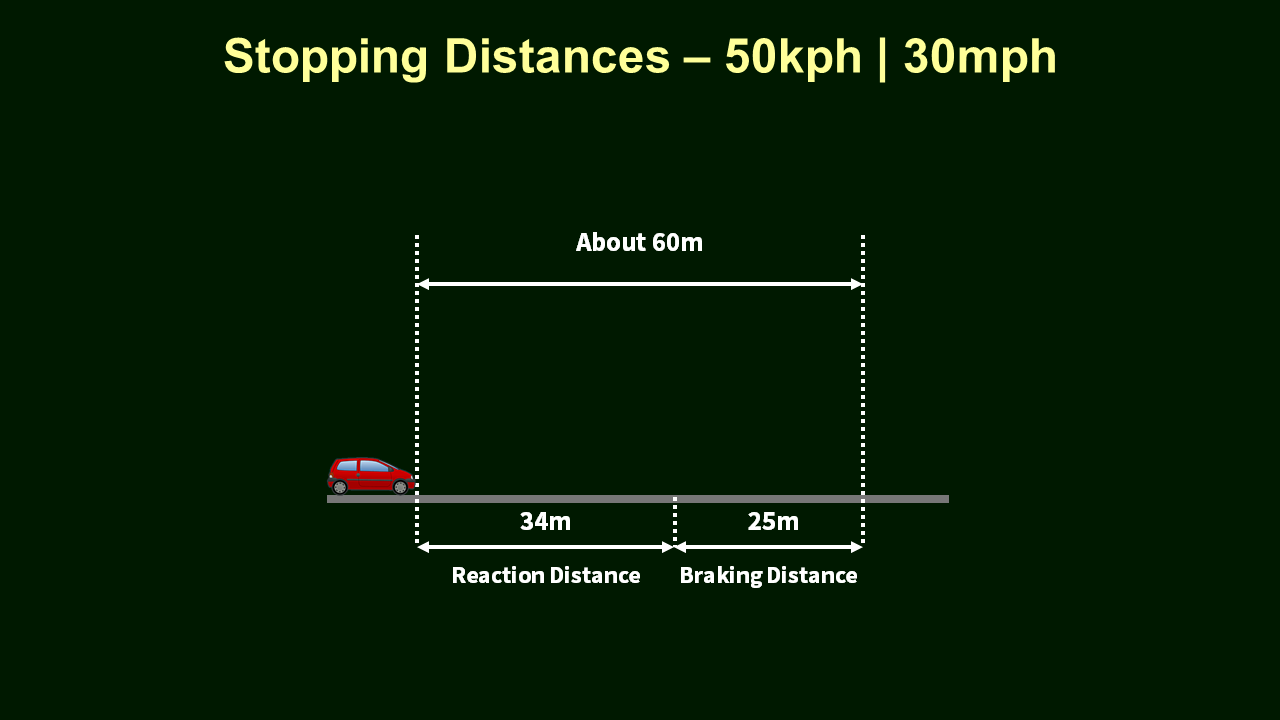
The answer's quite complicated because it's a combination of risk modelling from published traffic accident data, the Abbreviated Injury Scale, Occam's Razor (have the least assumptions), running what's called sensitivity analysis, and ease of use.
Perhaps most importantly, 'a difference is only a difference if it makes a difference'. We don't have the data to confirm this because it doesn't yet exist, but we suspect VALID's risk model is over-valuing the consequences in some parts of traffic because of the safety measures that cars have to protect the occupants during accidents; the model's erring on the side of caution for consequences. However, does this matter? Does it make a difference to the actual risk output?
To test this, in the model, we can drop the consequences one or two orders of magnitude in each scenario, with sensitivity analysis, and see how much a difference that makes to the risk. Doing this, it's clear red risks aren't turning green. That means the duty holder is still going to do something to reduce the risk even if the consequences are overvalued.
Cranking up the numberwang to try and model trees and traffic more accurately is not only fraught with mathematical problems and increased uncertainty, but it's not going to make a difference to the decision-making of the assessor or duty holder. It would also add another layer of complexity to the risk model when it comes to decision-making where you combine traffic and people in high-use occupancy. So why try to do it?
An analogy we've used is that we're looking at a big risk picture, and in one part of the canvas that's dealing with traffic and consequences it's a bit blurry. We could get down on our hands and knees with a magnifying glass and spend ages trying to make that consequences part a bit sharper. But when we've finished and taken a step back to admire our work, the risk picture isn't noticeably different.